Multimodal Learning
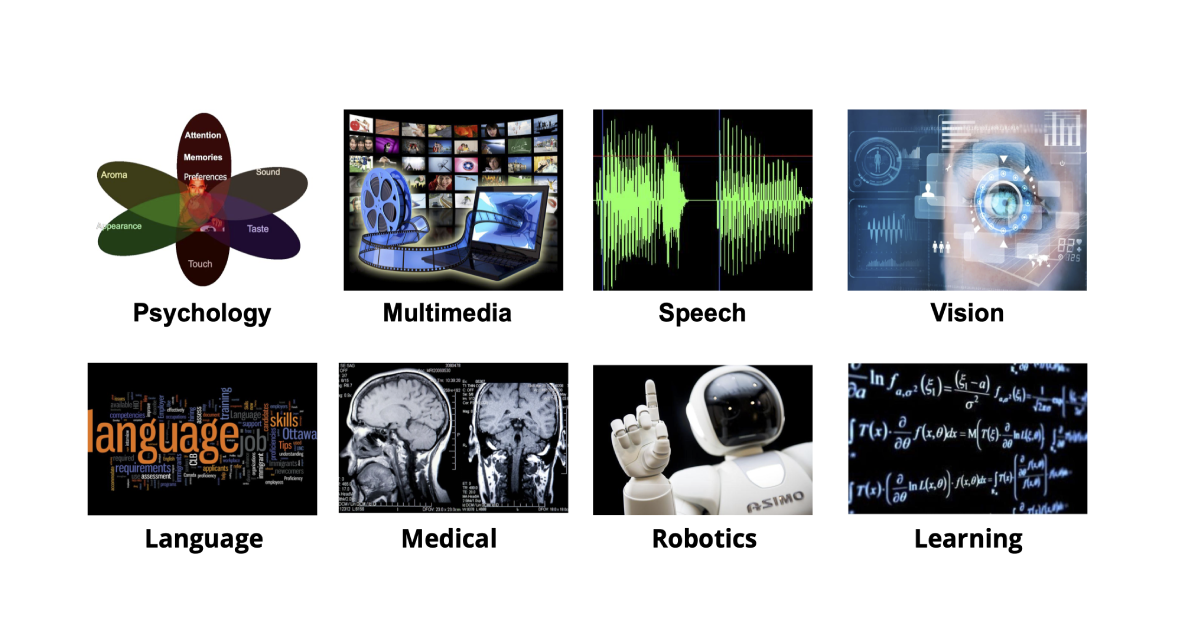
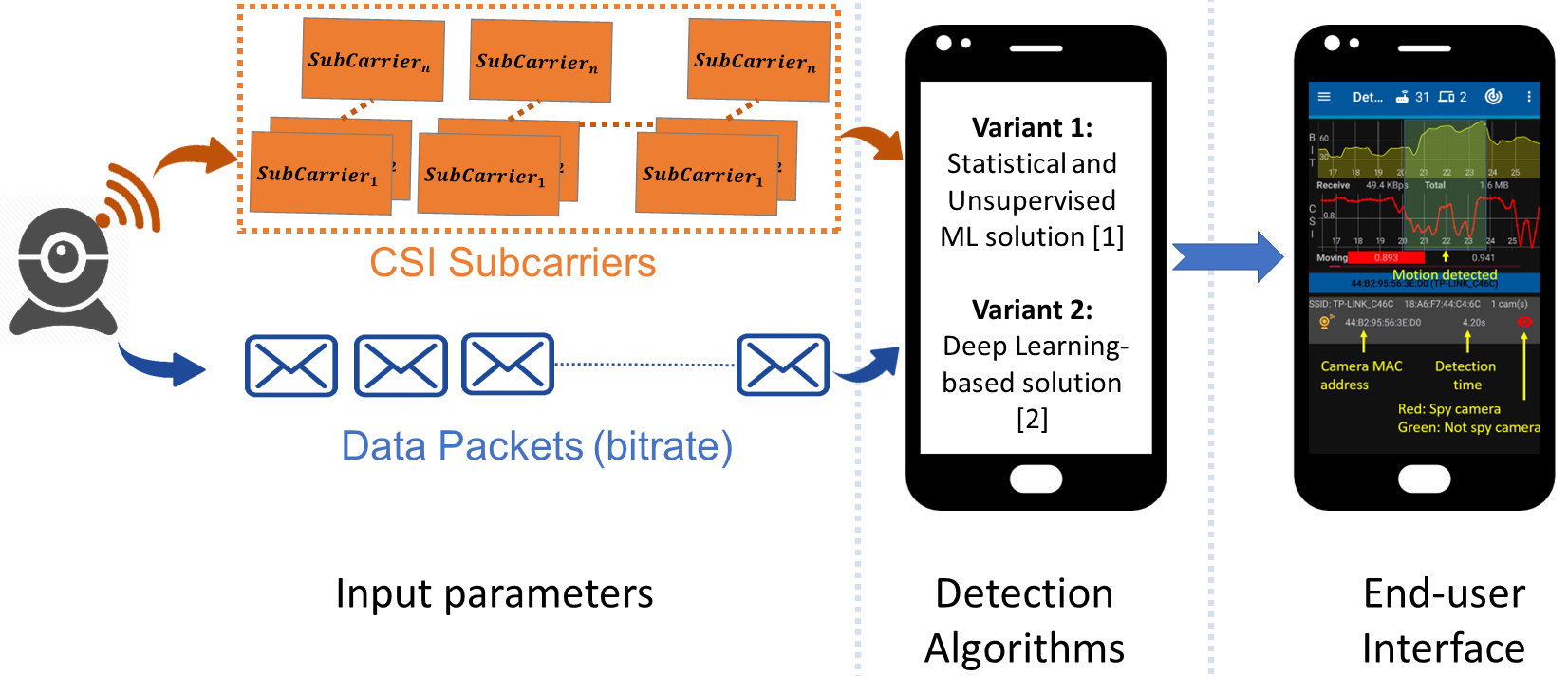
- • Digital Signal Processing (e.g., fourier transforms, signal filtering, in-band noise removal, etc).
- • Discreet Placement: CSI:DeSpy can be discreetly placed anywhere in the target space.
- • User-Friendly: No need for users to carry additional devices or perform preset actions.
- • Multipath-Rich Environment Resilience: Robust detection in challenging indoor settings.
- • Real-Life Testing: Thoroughly assessed in diverse scenarios to ensure effectiveness.
2. Contactless Driver Stress and Fatigue Detection
In our research, we frequently gather data from various sensors. As illustrated in the figure above for fatigue detection, we employ the mmWave Radar to non-invasively collect physiological indicators like heart rate (HR) and respiration from the driver, while also utilizing a video camera to capture signs of driver fatigue. In addition to these sensors, we may use some wearables such as Polar H10 sensor for contact-based heart reading as a ground truth, and an E4 wrist wearable device for supplementary information such as skin conductance measurement. The data collected from these various sensors come in different forms (e.g., morphologies and data patterns, etc.). However, the primary goal, which is extracting fatigue information, remains consistent across all of them. To extract fatigue information from the multimodal data, we need to integrate the data from various models in a manner that combines their unified features to yield more precise and valuable insights. The unified features are then provided to a deep learning model to anticipate the fatigueness of the driver. If you are open to working in this field, having a foundational understanding of the following domain would be beneficial:
- • Digital Signal Processing (e.g., fourier transforms, signal filtering, in-band noise removal, etc).
- • Computer Vision (e.g., face detection, valance and arousal analysis, dilated pupil detection etc.)
- • Data Fusion (e.g., feature, information, contextual fusion etc.)
- • Deep Learning (e.g., deep multimodal learning, transfer learning etc.)
Publications
-
Proceedings of the ACM on Interactive, Mobile, Wearable and Ubiquitous Technologies 6.2, 2022
- CSI: DeSpy: Enabling Effortless Spy Camera Detection via Passive Sensing of User Activities and Bitrate Variations
- Muhammad Salman, Nguyen Dao, Uichin Lee, Youngtae Noh
-
Adjunct Proceedings of the 2023 ACM International Joint Conference on Pervasive and Ubiquitous Computing and Proceedings of the 2023 ACM International Symposium on Wearable Computers (To appear)
- Poster: A Contactless and Non-Intrusive System for Driver's Stress Detection
- Muhammad Salman, Hyunkyu Jang, Youngtae Noh, Seungwan Jin, Dayoung Jeong, Hoyoung Choi, Kyungsik Han, Hyangmi Kim
Research Participants
-
- Postdoctoral Researcher
- Muhammad Salman
-
Research Interest
- Spy camera detection
- Occupancy monitoring
- Wireless Networks and SDN
- Bufferbloat mitigation
- Contactless Stress Detection
-
- Undergraduate Researcher
- Hyunkyu Jang
-
Research Interest
- Wireless communication
- Smart Grid
- 다음글 : Digital Healthcare
- 이전글 : Energy and Data Science