Energy and Data Science
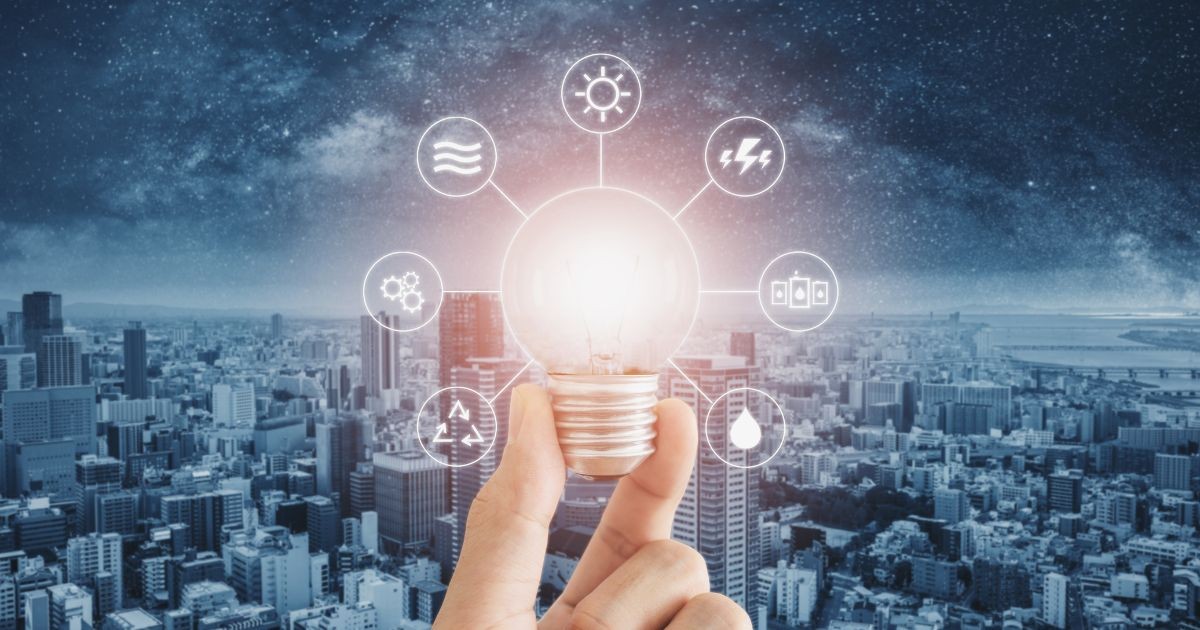
In the nexus of energy and data science, energy behavior emerges as a cornerstone for shaping sustainable futures. Energy behavior can be defined as the entirety of human actions that influence how energy is harnessed and consumed, encompassing everything from our choice of energy-efficient technologies to our daily consumption habits and the cognitive processes that drive these decisions. Data science plays a pivotal role in understanding these behaviors by extracting intricate patterns from an avalanche of data sourced from smartphones, wearables, and energy consumption metrics and providing granular insights into energy consumption habits. We develop technologies that employ digital phenotyping to discern energy consumers' behavioral patterns, inducing energy-saving habits among users. We aim to usher in an energy-efficient lifestyle that stands in harmony with our global aspirations for carbon neutrality.
1. EV Departure Time Prediction for DFC BMS algorithm
EV (Electric Vehicle) face challenges of battery degradation as the capacity diminishes with successive charge and discharge cycles, leading to reduced vehicle range. To address this, we develop an AI-driven DFC (Delayed-Full Charging) BMS (Battery Management System) algorithm that predicts EV unplug time, to minimize the duration batteries remain at full charge. We base our research on the idea that the unplug time is determined by departure time of EV, which is inherently tied to human behavior. By utilizing lifelogging data through digital phenotyping, we capture the intricate aspects of departure behaviors across variable scenarios. We hope to reduce replacement and social costs related to waste battery disposal, and accelerate the adoption of battery-based vehicles and energy storage systems.
2. Community Energy Management System (CEMS)
The Community Energy Management System (CEMS) is an innovative venture designed to streamline and fine-tune energy utilization across our campus, encompassing research facilities, administrative buildings, and residential areas. Recognizing the unique energy consumption patterns inherent to each building type, CEMS is developed to align its management strategies with the specific energy signatures of each establishment. Central to our academic exploration in the realm of CEMS is the integration of fast demand response (fast DR) mechanisms. The methodology commences with the identification of pivotal, controllable loads that directly influence campus energy consumption. By leveraging very short-term load forecasting techniques differentiated by time granularity, we can respond to and manage energy demands in an immediate and dynamic fashion. Our research can support user satisfaction and experiential input while stressing fast, efficient energy management. As a result, we cannot forge ahead with improvements in energy efficiency while also ensuring the overall comfort and well-being of users.
3. Quantified-self For Energy Efficient Lifestyle
Household energy consumption is on the rise globally, with Europe and the U.S. seeing residential sectors accounting for about 30% of their total energy usage. Similarly, in China, households are now responsible for 11% of the nation's energy consumption. This growing demand intensifies global climate challenges and escalates carbon emissions. Addressing these issues requires boosting energy efficiency and managing energy demand concurrently. In our research, we focus on developing foundational technology to model energy consumption behavior by utilizing life-logging data from mobile devices and smart meters. Our study can provide personalized energy-saving strategies based on individual energy consumption patterns.
Related Articles
KENTECH 송주현·노영태 교수팀, 삼성미래기술육성사업 지원과제 선정 - 전기신문 (2022.10.31)
삼성미래기술(23.01~25.12)
Publications
-
Ubicomp
- Poster: Departure Time Prediction Using Smartphone Data for Delayed-Full Charging BMS Algorithm
- Yonggeon Lee, Woojin Song, Juhyun Song, Youngtae Noh
- Adjunct Proceedings of the 2023 ACM International Joint Conference on Pervasive and Ubiquitous Computing and Proceedings of the 2023 ACM International Symposium on Wearable Computers
Research Participants
-
- Integrated PhD Student
- Yonggeon Lee
-
Research Interest
- Sensor Data Science
- Energy Behavior Modeling
- Energy Management System
- Digital Therapeutics
-
- Undergraduate Researcher
- Doohyun Kim
-
Research Interest
- Smart Grid
- Multimodal AI
- 다음글 : Multimodal Learning
- 이전글 : Networking